It’s the beginning of a new year, typically the time people make resolutions. A popular one is to improve their health. Besides joining a gym, they begin wearing devices that monitor and record their performance, like a Fitbit. The device gives real-time feedback on how they are doing and if they need to adjust their performance. Imagine a Fitbit for an entire system, like a giant wind-turbine farm.
Well, that is what OR assistant professor Sara Shashaani, along with Eunshin Byon from the Industrial and Operations Engineering Department at the University of Michigan, is researching thanks to a three-year grant from the Engineering Directorate of the National Science Foundation (NSF). “A peer-reviewed grant from the NSF indicates that other researchers and government leaders think the research is important,” said Julie Swann, ISE department head.
What is a Digital Twin?
Their research focuses on using big data to tune existing digital twins. So, what is a digital twin? “A digital twin is a virtual representation of a physical system, a collection of computationally expensive simulation models based on the physics of the system,” explained Shashaani. “It is a concept gaining increased popularity these days for its potential to revolutionize several fields, such as renewable energy production.”
For example, researchers will equip a wind turbine with sensors to record data about the different parts of the turbine’s performance. That data is sent in real-time to a processing system and then to the digital copy of the turbine.
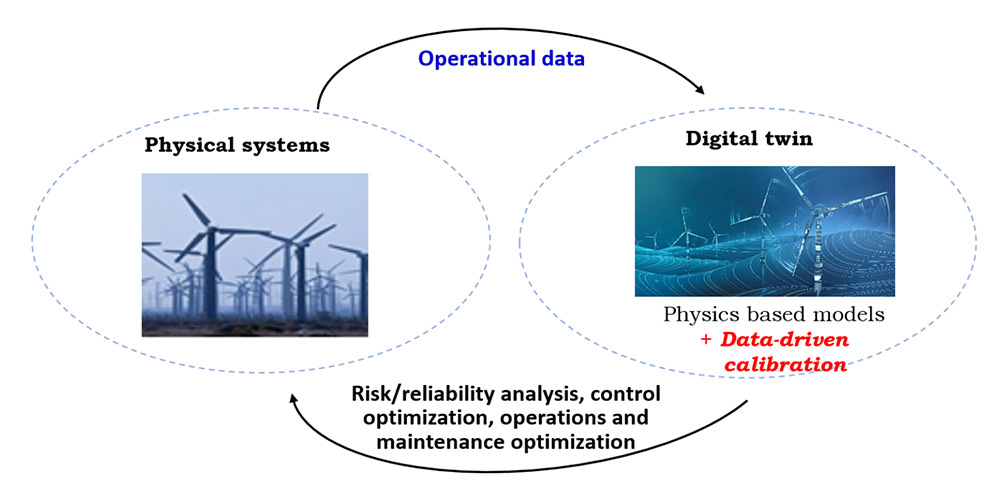
With this data, the virtual model can run multiple simulations to study various performance issues and generate valuable insights on improving the turbine’s output. These insights are then applied back to the turbine.
The Research
One of the challenges with digital twins is processing the vast amounts of data collected from the physical system, even with cutting-edge computing capabilities. For example, the year-long data of a single wind farm (500,000+ measurements) would require more than 8,000 hours of run time for calibrating only one parameter.
“This project aims to develop efficient and robust methods for calibrating digital twins using big observational data,” said Shashaani. She will develop stochastic optimization methods integrated with sampling techniques to identify small subsets of the large data containing the same amount of information. This approach will help with speed but will also provide reliable calibrations. Digital twins will only be effective if they are well-calibrated.
The Impact
“The outcome of this project will enable the effective usage of digital twins for real-world decision-making with robust use of large datasets in a short time,” explained Shashaani.
While she and her collaborators will show how their methods can improve the performance of wind farms and building heating and cooling as a prototype, the impact of their research will go beyond just energy applications. “It can benefit supply chain management, healthcare, and manufacturing systems, by establishing a digital counterpart of reality via cost-effective yet accurate simulation,” shared Shashaani.
Collaboration with the National Renewable Energy Laboratory and Risø National Laboratory in Denmark will allow this project’s outcomes to have a high chance of being implemented to improve current systems. The project has a vital educational component, such as summer camps and undergraduate researchers’ recruitment.
“We all celebrate when our faculty, especially our newer faculty, obtain a grant from the NSF,” said Swann. “Dr. Shashaani is developing the exciting methodology to solve significant problems for humankind.”
The Team
Want more details about Shashaani’s NSF grant? Go to the NSF website.

Sara Shashaani
Sara Shashaani joined the Department of Industrial and Systems Engineering as an assistant professor in January 2019. Before joining the NC State faculty, she was a postdoctoral fellow at the Department of Industrial and Operations Engineering at the University of Michigan, where she worked on designing and improving probabilistic predictive models specifically used for hurricane-induced power outages, with challenges in highly imbalanced datasets and a large set of explanatory variables. Her dissertation research in the area of derivative-free simulation optimization awarded her a Ph.D. degree in Industrial Engineering from Purdue University in 2016. Besides her research, she is passionate about activities that target the environment, community wellness, and science communication.

Eunshin Byon
Eunshin Byon’s research interests include reliability evaluation, fault diagnosis/condition monitoring, predictive modeling and data analytics, and operations and maintenance decision-making for stochastic systems. Her recent research focuses on uncertainty quantification of stochastic systems using stochastic simulations, reliability analysis and improvement of large-scale, interconnected systems with applications to renewable power systems and manufacturing processes. She is a member of IIE, INFORMS, and IEEE.
- ebyon@umich.edu
- 734.764.6565
- Byon’s personal website